Understanding the Significance of a Data Labeling Platform
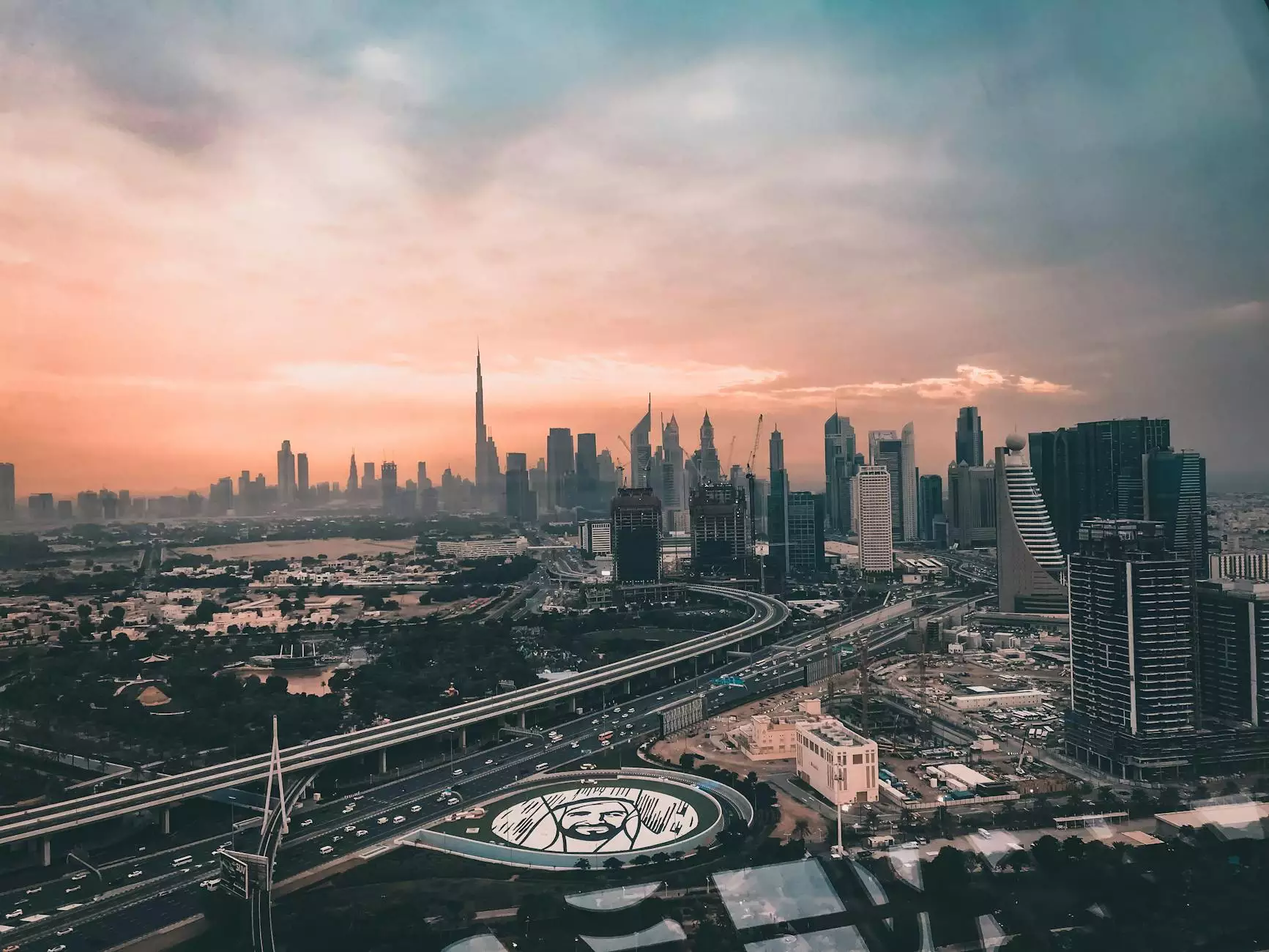
In the rapidly evolving landscape of artificial intelligence (AI) and machine learning (ML), the need for accurate and reliable data labeling platforms has never been more crucial. As businesses increasingly leverage AI to drive innovation and efficiency, the role of precise data annotation emerges as a cornerstone of successful AI implementations.
What is a Data Labeling Platform?
A data labeling platform is a specialized software tool designed to aid in the process of annotating data—a vital step in preparing data for machine learning models. By systematically tagging data, whether it is images, texts, or audio, these platforms enable computers to learn from the annotated information, thus enhancing the performance of algorithms.
Key Features of Data Labeling Platforms
When exploring a data labeling platform, you will encounter a range of features designed to streamline the annotation process and improve the quality of labeled data. Key features include:
- User-Friendly Interface: An intuitive layout that simplifies the annotation process for teams.
- Collaboration Tools: Features that facilitate teamwork, allowing multiple users to annotate concurrently.
- Automated Annotation: Leveraging AI to pre-label data, speeding up the labeling process and increasing consistency.
- Quality Assurance Mechanisms: Systems to validate labeled data and ensure high accuracy rates.
- Custom Templates: The option to create custom annotation templates specific to unique project requirements.
- Integration Capabilities: Supporting seamless integration with other software tools and workflows.
The Importance of Data Annotation in AI Development
Data annotation is integral to training machine learning models effectively. Without properly labeled data, models struggle to discern patterns and make accurate predictions. Here are several reasons why data annotation is indispensable:
1. Enhancing Model Accuracy
When data is labeled correctly, it provides the foundation for machine learning models to learn effectively. The more accurate the annotations, the more reliable the model predictions will be. For businesses relying on AI, this can translate into significant operational benefits.
2. Supporting Diverse Use Cases
A robust data labeling platform can handle various data types, ranging from images to healthcare insights, enabling application across multiple industries. From autonomous driving to natural language processing, accurate labels are vital in empowering diverse use cases.
3. Reducing Time and Cost
Utilizing an efficient data labeling platform can significantly reduce the time and costs associated with manual labeling. By automating sections of the process and enabling better collaboration, companies can accelerate project timelines and optimize resources.
How to Choose the Right Data Labeling Platform
Selecting the right data labeling platform is crucial for maximizing the efficacy of your data annotation process. Consideration of several factors can make a significant difference:
1. Assess Your Needs
Identify your specific project requirements and the type of data you intend to label. Different platforms may specialize in various formats or annotation techniques. Determining your needs upfront will help narrow your options.
2. Evaluate Platform Features
Ensure that the platform offers the essential features outlined previously. Additionally, look for advanced capabilities such as AI-assisted annotations or support for complex labeling tasks.
3. Consider Scalability
As your projects scale, your data labeling needs might evolve. Choosing a scalable platform that can grow with your business is vital to accommodate fluctuating volumes of data and changing project scopes.
4. Check Customer Support
Reliable customer support can make a significant difference, especially during critical moments when you may need technical assistance. Look for platforms known for their responsive customer service and comprehensive user support resources.
5. User Reviews and Case Studies
Researching existing user reviews and case studies can provide insight into the platform's performance and the experiences of other businesses. Look for feedback that highlights strengths, weaknesses, and any potential red flags.
Examples of Successful Use Cases
To better understand the capabilities of a data labeling platform and how they can drive success, let us take a look at several real-world examples:
1. Autonomous Vehicles
Companies developing self-driving technology rely heavily on precise data labels for training their algorithms. By utilizing advanced labeling platforms, they can annotate vast amounts of driving data, including object detection and lane segmentation, ultimately leading to safer autonomous navigation.
2. Healthcare Analytics
In healthcare, accurately annotated datasets are crucial for predictive analytics, diagnostics, and improving treatment outcomes. Data labeling platforms assist in tagging medical images, patient notes, and research data, contributing to enhanced medical AI solutions.
3. E-commerce Personalization
E-commerce platforms utilize annotated data to personalize shopping experiences. By effectively labeling customer interaction data and product images, businesses can offer tailored recommendations, driving customer engagement and boosting sales.
The Future of Data Labeling Platforms
As the field of AI continues to progress, the data labeling landscape is expected to transform in several key ways:
1. Increased Automation
Machine learning will increasingly automate labeling tasks, reducing manual effort and enhancing efficiency. This shift toward AI-assisted annotation will further elevate the accuracy and speed of data labeling platforms.
2. Enhanced Data Security
With growing concerns around data privacy and security, future labeling platforms will likely place a stronger emphasis on safeguarding sensitive data, ensuring compliance with regulations while maintaining high labeling quality.
3. Tailored Solutions
As industries continue to evolve, there will be a demand for more customized data labeling solutions that cater specifically to niche requirements and unique data types.
Conclusion
In conclusion, a robust data labeling platform is not just a utility but a strategic asset that can significantly influence the success of AI projects. By understanding the features, benefits, and the critical role of data annotation, businesses can make informed decisions when selecting their data labeling tools. As the landscape continues to evolve, those who stay ahead of the curve by choosing the right platform will undoubtedly thrive in the age of AI.
About Keylabs AI
At Keylabs AI, we provide cutting-edge solutions for data annotation, ensuring that your AI initiatives are backed by high-quality, accurately labeled data. Our data annotation platform is designed to cater to the specific needs of businesses looking to leverage AI effectively. With a commitment to quality and efficiency, Keylabs AI is here to support your journey into the future of technology.
© 2023 Keylabs AI. All rights reserved.